You Are What You Eat - AI Alignment Requires Understanding How Data Shapes Structure and Generalisation
Best AI papers explained - A podcast by Enoch H. Kang - Tuesdays
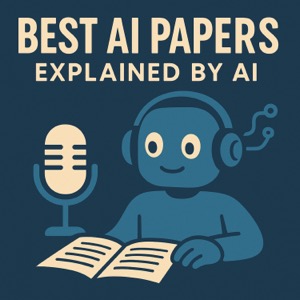
Categories:
This academic paper, "You Are What You Eat - AI Alignment Requires Understanding How Data Shapes Structure and Generalisation," posits that achieving AI alignment necessitates understanding the relationship between the data distribution used for training and the resulting internal structure and generalization patterns of the model. The authors argue that traditional testing methods are insufficient because models with similar training performance can generalize differently based on their internal workings, which are deeply influenced by the training data. They advocate for developing a mathematical science of AI alignment by studying how data patterns shape internal model structures and subsequently impact generalization, suggesting that current alignment techniques primarily rely on indirectly shaping model behavior through data. The paper explores existing research supporting this connection, including the emergence of algorithmic structure in neural networks and principles from singular learning theory, while also discussing the challenges posed by inductive biases, dangerous patterns in real-world data, and distribution shift. Ultimately, the authors contend that a fundamental understanding of this data-structure-generalization pipeline is crucial for building robust and reliable aligned AI systems, moving beyond empirical approaches towards a more rigorous engineering discipline.keepSave to notecopy_alldocsAdd noteaudio_magic_eraserAudio OverviewmapMind Map