Predictions as Surrogates: Revisiting Surrogate Outcomes in the Age of AI
Best AI papers explained - A podcast by Enoch H. Kang - Fridays
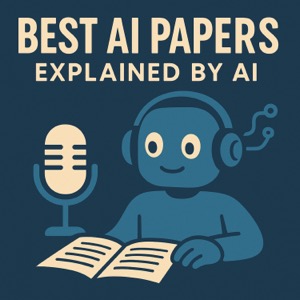
Categories:
This paper explores a new method for statistical inference in the age of AI, focusing on how predictions from large pre-trained models can serve as efficient surrogates for costly or difficult-to-obtain outcomes. Drawing a connection to the established field of surrogate outcome models in biostatistics and economics, the authors propose recalibrated prediction-powered inference (RePPI). RePPI is presented as a more efficient approach than existing methods by learning an optimal "imputed loss" function, which helps to improve accuracy and efficiency even when the predictions are imperfect. The paper theoretically analyzes the benefits of recalibration, particularly in scenarios involving modality mismatch, distribution shift, and discrete predictions, and demonstrates its practical advantages through real-world applications.