DRAFT: Self-Driven LLM Tool Mastery via Documentation Refinement
Best AI papers explained - A podcast by Enoch H. Kang
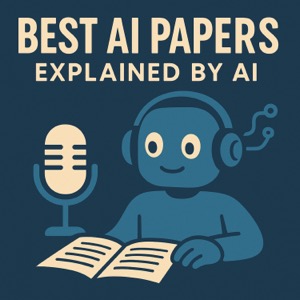
Categories:
This paper introduces tool learning, where large language models utilize external tools to enhance their capabilities in complex tasks. A key challenge in this area is the quality of tool documentation, which often suffers from incompleteness, redundancy, or inaccuracies. To address this, the paper proposes DRAFT, a self-driven iterative framework that enables LLMs to automatically improve tool documentation through exploration and feedback. This framework includes experience gathering, learning from experience, and documentation rewriting phases, along with mechanisms to ensure diverse exploration and adaptive termination. Experimental results demonstrate that DRAFT significantly enhances tool documentation, leading to improved performance in tool usage across various language models.